Asset Allocation with Generative AI Part II: Factor Investing in Asset Allocation
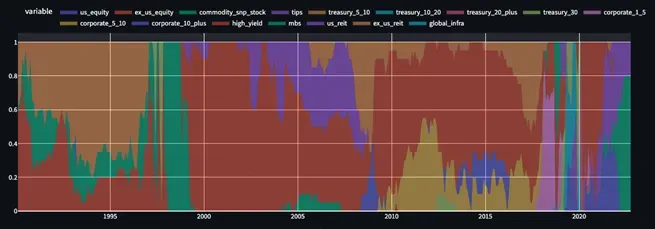
Summary of the talk given at the 6th Annual Korea Quantitative Investment Conference 2022
The series on the topic ‘Asset Allocation with Generative AI’ consists of the following 3 sections.
Part 1: Motivation behind Generative Approaches
Part 2: Factor Investing in Asset Allocation
Part 3: Generative Factor Model
In this section, we will explore the recent advances in Generative AI and the impact it will have on quantitative investment
Factor Investing in Asset Allocation
Why does Asset Allocation need AI?
1. There are too many asset classes
There are diverse asset classes ranging from Equity (U.S., Emerging, International), Bonds (government and corporate with different durations), Gold, Commodity (Corn, Copper, Metal), REITs, and Global Infrastructure.
2. There are many macroeconomic data
There are diverse macroeconomic data ranging from GDP, CPI, Yield Curve, and many more — Federal Reserve Economic Data (FRED) provides more than 740,000 data. Each data are sourced from different data providers and pre-processed in a specific manner.
3. Static / Rule-based Asset Allocation is limited
Due to a limited understanding, investment managers tend to use only a handful of macroeconomic data. Consequently, they deploy models dependent only on a few variables that are prone to a volatile market.
Complemented by a data infrastructure that covers information on all types of asset classes and pre-processes vast macroeconomic data in scale, AI models can fully utilize the information provided by the rich data. The question then really leads to how models can be trained generatively.
Factor Investing as a Starting Point
We believe that factor investing is an ideal starting point to think about how generative models can be trained. Before we delve into a rather unprecedented approach to applying factor models in asset allocation, I will first discuss factor investing in equity-level portfolio management which is more familiar.
At an equity level, a factor is defined as a combination of company financials that is often understood as a source of underlying risk. In many different cases, a factor is also defined as a source of alpha, a source of excess returns relative to the market returns usually represented by S&P500 and NASDAQ Composite Index. Take PER for instance — it is a combination of company fundamentals market value and net income, and mathematical operator division. The idea is to calculate the PER of all the companies in the investment universe and take the long-short position of each company according to the quantile that its PER falls onto.
We thought that a similar approach could be taken to tackle a multi-asset allocation problem. If a factor at an equity level is defined as a combination of fundamentals, can we not define a factor at a multi-asset level as a combination of macroeconomic variables? Misery Index is a good example — the index is calculated by summing the annual inflation rate with the season-adjusted unemployment rate to measure the degree of economic distress felt by an average person. Our premise is that such a combination of variables can play a prominent role in forming macroeconomic factors to generate multi-asset allocation investment strategies.
Multi-Asset Factor Investing
Akros defines the formulation of macroeconomic alphas and multi-asset allocation strategies as multi-asset factor investing. A procedure that we follow for finding a macroeconomic factor is as follows:
1.Prepare and formulate a macroeconomic factor. Figure 1 shows an example of a macroeconomic factor relating to M1.
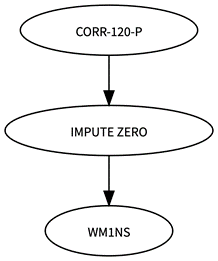
2. Calculate the value in the time series. Figure 2 below shows a time- series plot of the sample macroeconomic factor.
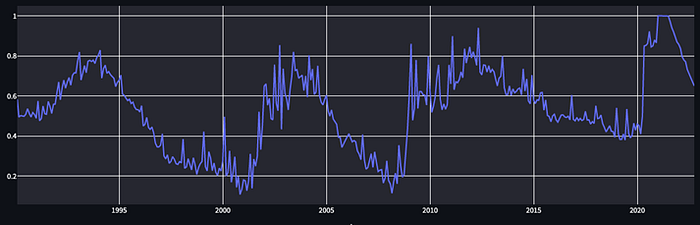
3. At the end of each month, take the macroeconomic factor as a benchmark in order to allocate the weight of each asset (-1, 1) in the investment universe to maximize the correlation between the allocated asset returns and the macroeconomic factor.
4. Re-balance at the end of each month to obtain a long-short dynamic multi-asset portfolio. Figure 3 below shows the dynamic changes in the weight of each asset class.
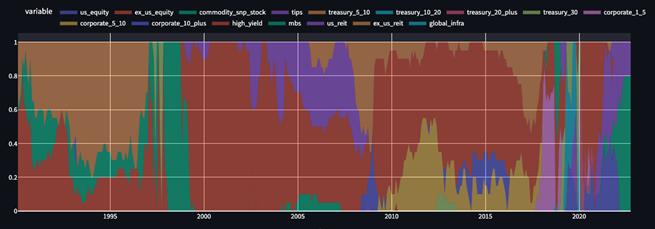
Although the concept of multi-asset factor investing is unprecedented and experimental in the sense that it has not been analyzed in academia before, we believe that it is a meaningful attempt to reflect the macroeconomic impact on asset allocation. Figure 4 below shows examples of ‘good’ factors that we have found using our approach of combining various macroeconomic variables. The first factor on the left-hand side, for instance, is formulated by multiplying term spread with federal net outlays and dividing by GDP.

A ‘good’ macroeconomic factor that we term ‘Macroeconomic Alpha’ is defined by setting particular criteria for the performance of the multi-asset portfolio returns that it yields. In our case, we have defined the macroeconomic alpha as a factor that yields portfolio returns with HAC-adjusted t-statistic in the recent 20 years greater than the value of 3. The criteria for a macroeconomic alpha can certainly be adjusted depending on the goal that the portfolio seeks to achieve to satisfy varying investor needs.
A broader pipeline of multi-factor investing that converts macroeconomic factors into the final portfolio construction is highlighted by the diagram in Figure 5. The first step is to generate macroeconomic factors in scale. Of all the formulated macroeconomic factors, we elicit macroeconomic alphas using the performance criteria that we have selected. The third step is to combine all the macroeconomic alphas that we have found into one single alpha. The final step is to construct a final portfolio using the combined macroeconomic alphas.
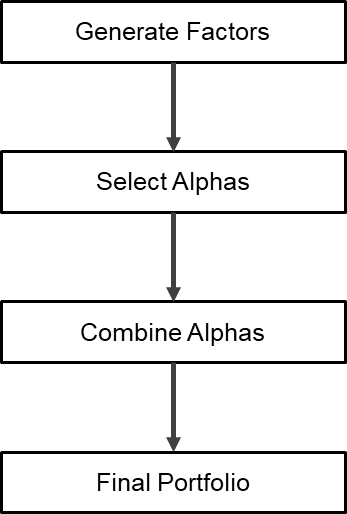
The ultimate goal of the pipeline is to maximize the performance of the final portfolio. There are several points to consider as we strive to achieve the feat:
- We need to find more macroeconomic alphas.
- We need to find greater macroeconomic alphas.
- We need to find alphas at a faster rate.
- We need to find alphas efficiently at a manageable cost.
Unfortunately, none of these points can be achieved using human-based approaches that would include intuitive guesses and numerous trials & errors at an inefficient rate. That’s why we at Akros have decided to develop a Quantitative Generative AI model to find numerous, high-quality macroeconomic alphas efficiently in scale (Part 3).
Part 3: Generative Factor Model
Thank you for your time and for those who are interested in learning more about Akros Technologies, please contact us via email
Also, here are a few links you could refer to!