Asset Allocation with Generative AI Part I: Motivation behind Generative Approaches
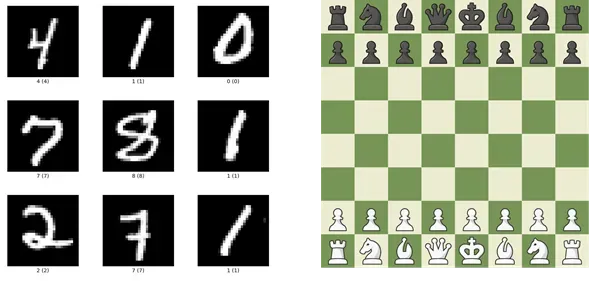
Summary of the talk given at the 6th Annual Korea Quantitative Investment Conference 2022
The series on the topic ‘Asset Allocation with Generative AI’ consists of the following 3 sections.
Part 1: Motivation behind Generative Approaches
Part 2: Factor Investing in Asset Allocation
Part 3: Generative Factor Model
In this section, we will explore the recent advances in Generative AI and the impact it will have on quantitative investment
The Motivation behind the Talk: “A Giant Leap”
Many of the advances in quantitative investment have been inspired by scientific and technological innovation. The Black-Scholes equation that we often use in options trading actually stems from Quantum Mechanics. Many of the techniques that we use in modern portfolio theory whether it be mean-variance optimization or capital asset pricing are the application of statistics and linear algebra. And as such, I am excited by the innovation in technology and the impact that it will have on quantitative investment.
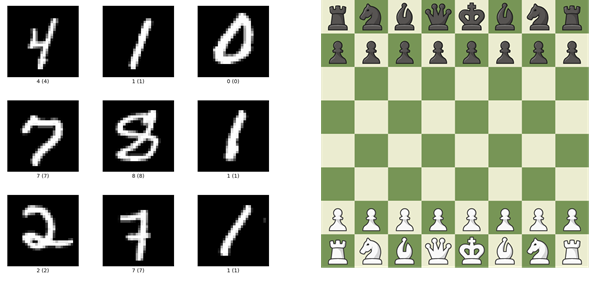
Over the last few years, we have witnessed an amazing breakthrough, a giant leap if you will, in the domain of AI. Traditional AI models were initially developed to solve relatively simple tasks like digit recognition using the MNIST dataset and play games like chess within a hard set of rules as shown in Figure 1. Models like AlphaGo tended to be domain-specific, meaning that there were separate models each specialized in particular tasks like chess, go, and Atari trained using the corresponding datasets. The recent progress in the field, however, has leaped drastically in the last few years. Modern AI models are often domain agnostic and are able to generalize over multiple complicated tasks. AlphaGo has evolved into AlphaZero capable of playing chess, go, and Atari all simultaneously at a super-human level.
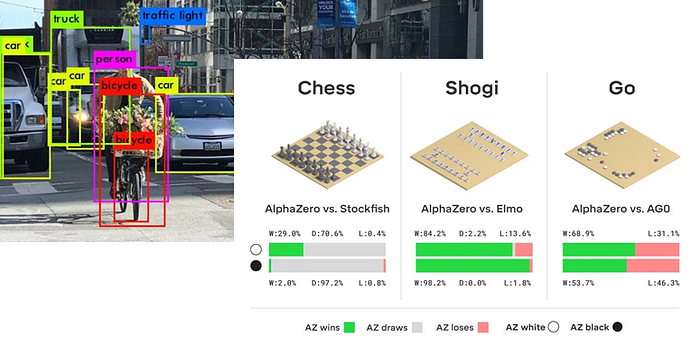
When we carefully analyze the recent breakthrough, there have been significant changes to the ways that machine learning and deep learning models are trained. Whereas traditional models have treated the data in a rather discretive way and have been trained data in a point-wise manner, recent models have learned to understand the data at a higher level including the distribution of the data as well as its meta-level properties.
The advance in the ways that they are trained means that the modern AI models now venture beyond simply learning and inferring from the given dataset to create and imaging a whole new unforeseen data. These models are what we call Generative AI. As shown by Figure 3, this is a development from the discriminative models that were used to solve simple tasks like image classification problems categorizing images to dogs and cats, to generative models that imagine an output from a query such as “Draw a dog in a house made of sushi.” Deep learning models like Dall-E 2 have gone further to accomplish tasks such as image generation (creating a new fashion) and image inpainting (generating additional painting to an existing image).
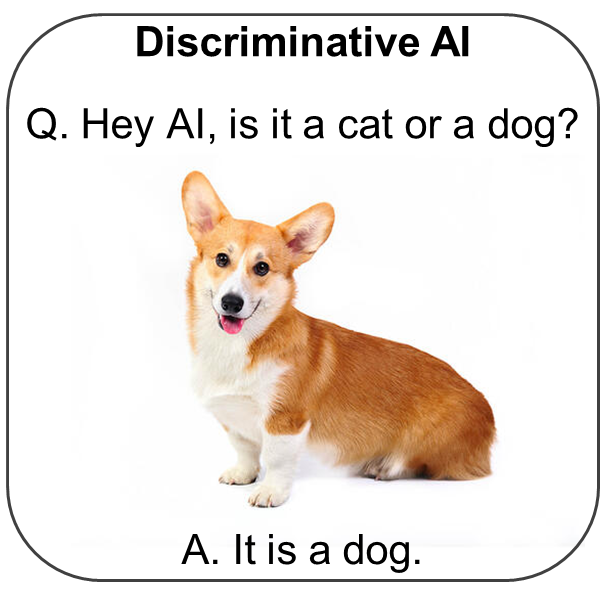
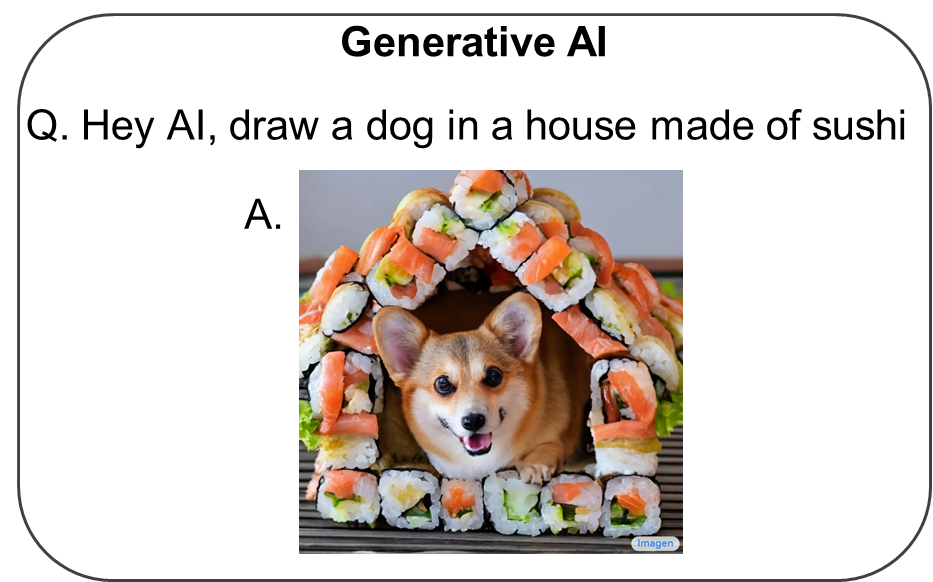
And as such, just like how scientific and technological innovation has already had a huge influence on the domain of text, images, and audio, through this talk, I want to address the impact that the recent advances to Generative AI will bring to quantitative investment.
AI in Quantitative Investment: From Discriminative to Generative
AI in Quantitative Investing has been Discriminative
Similar to other fields, discriminative AI models have been commonly used to make decisions in quantitative investment. Quantitative researchers often take a discriminative approach by formulating a regression problem that predicts the next 1-month returns of each company given the fundamental, price, and volume data. A selected number of companies predicted to give the highest returns or other metrics of choice are then used to create a portfolio. But there have been many problems with a such discriminative approach in quantitative investment.
Firstly, the problem formulation suggested above is likely to yield overfitting of the models leading to the prediction of the next 1-month returns using only the most recent returns in the past. With reference to Figure 4, this is to say that the prediction plot (highlighted in red) is simply equivalent to the 1-month shifted plot of the true returns (highlighted in blue). Since discriminative models are merely giving an output value of the most recent returns in the past, they are unlikely to provide important information for future investment decisions.
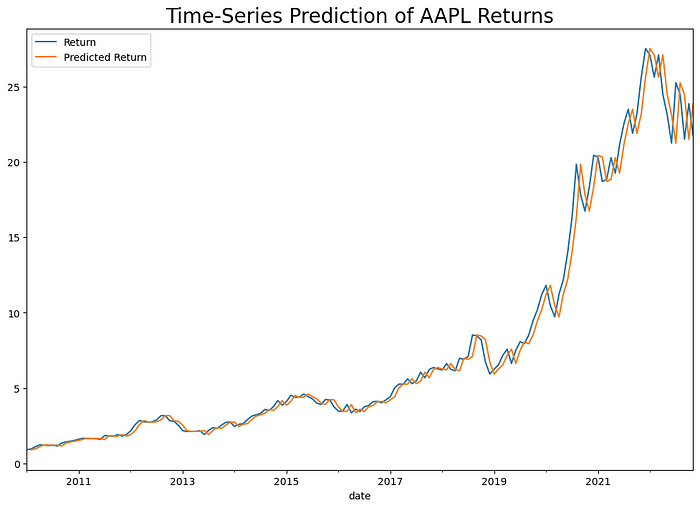
Secondly, there are problems related to feature importance. Neural Networks can opt to use a selected few features in making an inference from the given dataset regardless of the total number of features. Even though we can attempt to understand the level of importance of each feature using gradient-based methods like Grad-CAM and SHAP, there are additional difficulties in understanding how and why selected features are leveraged in making a prediction.
Finally, the discriminative approach in quantitative investment involves human decisions. Successful model discriminative inference on portfolio returns, for instance, leaves room for human managers to decide how the portfolios are selected given the information provided by the model output. Although there are potential benefits arising from combined human and model decisions, it means that the final decision is prone to human bias.
AI in Quantitative Investing will be Generative
The application of generative AI models will address many of the problems above. If we are able to successfully develop Quantitative Generative AI models, we will be able to input a query like this:
“Generate a strategy to achieve the annual dividend yield of 7%”

Quantitative Generative AI models can therefore learn to :
1. create an investment strategy with a particular goal of choice.
2. generate multiple investment strategies with different paths to reaching the goal.
There are multiple advantages that Quantiatitve Generative AI models can have over the traditional Quantitative Discriminative AI counterpart.
Firstly, the fact that the generative models can create an investment strategy itself means that an investment strategy can be generated free from human bias — it means that no human input is added in the final formulation of the investment strategy.
Secondly, the fact that generative models can create multiple strategies means that various diverse investment strategies with complementary properties can be generated. It is the very diversity of these strategies that can ultimately avoid overfitting and yield stable investment performance.
I will now move on to the main theme of this talk — how we at Akros Technologies are applying factor investing in asset allocation (Part II) and uncovering growth paths in a volatile market through Asset Allocation with Generative AI (Part III).
Part 2: Factor Investing in Asset Allocation
Part 3: Generative Factor Model
Thank you for your time and for those who are interested in learning more about Akros Technologies, please contact us via email
Also, here are a few links you could refer to!